Some Background
I am a faculty member in a technical communication program at a comprehensive research university. Recently, I have been inundated with questions, concerns, and critiques about the rise of augmentation technologies in writing and design processes, particularly generative artificial intelligence (AI) tools that support chat-based text generation and text-to-image production. I’m sure many UX researchers and designers face similar issues in their work. It remains unclear how generative AI should fit into existing workflow or design processes. Common questions include these:
- How does AI work? What can it do? Is it free?
- Is it cheating if I use AI to produce content?
- Who is responsible for the quality of AI-generated content?
- To what extent can I outsource my routine work to AI? In other words, what’s an acceptable threshold for using AI before it is considered too much?
Specific to UX is the value (cost and labor versus gains and effects) of generative AI in the research and design of user-centered products. Students in my UX courses are increasingly worried about the presence of AI and, consequently, the relevance of their developing skill sets in UX. Educators are growing wary about the presence of AI in the context of teaching and learning; many form partially informed decisions on academic policies for AI usage.
Factoring all these conditions, in this essay, I reflect on the intersection of UX education and AI, exploring the challenges and opportunities that arise from the integration of generative AI into the teaching of UX. I draw primarily on exchanges I made with UX professionals and educators, as well as my own reading of broader industry trends, to discuss institutional concerns, professional expectations, and ethical considerations related to the use of AI in UX education. I will also consider four scenarios of human-AI collaboration, proposed and discussed by a team of creativity researchers (Vinchon et al., 2023), as they pertain to UX and education in general.
Admittedly, my relationship with AI began only in the late 2010s when I explored immersive media experiences within the context of wearable technology and the Internet of Things (Tham, 2017, 2018). At that time, AI was mainly understood as a tool that could automate tasks and support decision-making activities with minimal human intervention. As I continued to engage with AI in various projects, including my collaboration with Tharon Howard and Gustav Verhulsdonck on a book, UX Writing: Designing User-Centered Content (2024), I began to consider more critically the implications of AI for technical communication and UX design. Following the release and popularization of ChatGPT, which marked a significant shift in public discourse around AI, I participated in numerous symposia and workshops focused on the implications of AI on writing pedagogy. My perspective, informed by human-centered design principles, expanded as I engaged in these discussions. This journey shaped how I understand AI today.
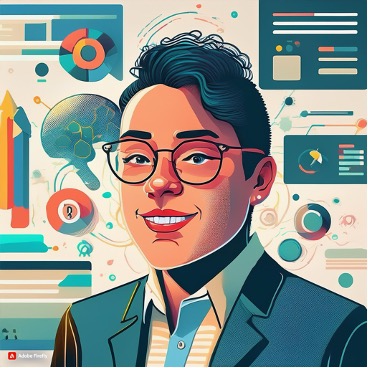
Figure 1. A text-to-image graphic generated with the author’s photograph as a composition reference (generated with Adobe® Firefly™ [Artificial intelligence system]).
The Hype
From my vantage point, generative AI has gained significant attention due to the rhetoric around its potential to revolutionize multiple fields (Ooi et al., 2023). Such rhetoric is founded on real experimentation. From fiction to news articles, generative AI tools speed up the content creation process with a high satisfaction rate (Noy & Zhang, 2023). Generative AI also composes art, music, and design that blends styles with surprising elements, often leading to new creative forms (Figure 1). Researchers and analysts consult AI for data analysis, hypothesis testing, and system modeling, which yields somewhat reliable results. Businesses and service providers use AI’s natural language processing abilities to personalize customer interactions and targeted marketing efforts, resulting in rather positive experiences (Hocutt, 2024).
The most crucial limitation of generative AI models is their reliance on the quality and quantity of the training data they are trained on. If the training data is biased, incomplete, or not representative, the AI’s outputs can reflect those issues, leading to biased, inaccurate, or unhelpful results. Second, generative AI does not understand the content it generates in a human-like way. It identifies patterns in data and replicates them, but it lacks true comprehension, meaning its outputs can sometimes be nonsensical or inappropriate. Unlike a person, AI often struggles with maintaining context over extended conversations or generating content that requires deep understanding of complex concepts, cultural nuances, or ethical considerations (Verhulsdonck et al., 2024). On the topic of ethics (discussed further in the “More on Ethics” section), AI can perpetuate and even amplify existing biases from its training data, leading to outputs that reinforce stereotypes or marginalize certain groups. Legal professionals are playing catch-up as generative AI outpaces regulatory frameworks, leading to uncertainties about legal responsibilities and ethical guidelines. So, when AI-generated content leads to harm or ethical dilemmas, it is often unclear who is responsible—the developers, the users, or the AI itself (Kalpokienè, 2024).
As AI develops, these real consequences are gradually addressed. Some problems are rectified. Regardless of the hype, AI gained a foothold in the world and continues to influence how we work. The implications of AI, professionally and institutionally, remain to be discussed.
Professional Expectations
From a career preparation standpoint, UX educators are rightfully concerned about the professional expectations that our students will face upon graduation (Schriver, 2023). These expectations are shaped by industry standards, technological advancements, and evolving workplace dynamics. Many popular sources claim that AI is rapidly becoming a key component of UX practice. Therefore, universities and institutions believe that students must be prepared to navigate this landscape with proficiency and confidence (Figure 2).
One of the primary professional expectations is that students will have basic proficiency with AI tools. This includes understanding how AI can be used in UX research and design and developing technical skills to work with AI-driven tools. However, proficiency alone is not enough. Students must also develop the confidence to make informed decisions about when and how to use AI in their work. This requires a deep understanding of the capabilities and limitations of AI and the ability to critically evaluate its impact on user experience.
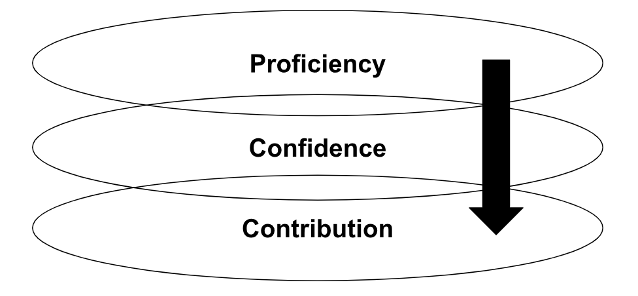
Figure 2. From knowing how to use AI tools to making contributions to AI development.
In addition to proficiency and confidence, students must also be prepared to contribute to future AI development. While not all students will pursue careers in AI development, they will likely encounter AI-driven tools and systems in their professional lives. As UX designers, they play a role in shaping the user experience of these tools, ensuring that the tools are accessible, ethical, and user-centered. This requires a solid foundation in both UX principles and AI technologies and a commitment to continuous learning in an ever-evolving field.
Institutional Responsibilities
Universities play a crucial role in shaping the values and resources available to students and educators as they navigate the integration of AI into education. The institution is the executive decision-making authority when it comes to handling AI. Given the functions and aforementioned ethical constraints of AI, universities need to be proactive in providing support.
One of the primary institutional concerns is the balance between student learning and wellness (Doukopoulos, 2024). Classrooms—whether physical or virtual—are spaces for promoting equity. Universities must consider the impact of AI on students’ mental health and cognitive load, particularly as they are exposed to new and potentially overwhelming technologies. The values that institutions uphold in this regard will influence how AI is integrated into the curriculum and how students are supported in their learning.
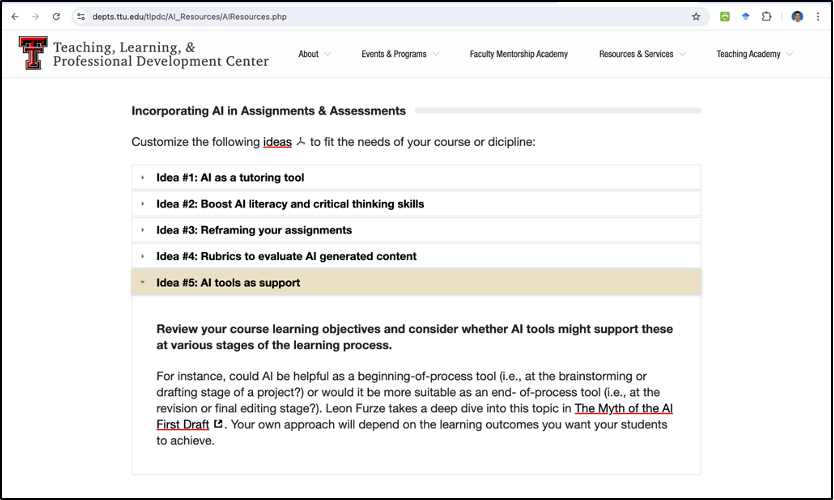
Figure 3. Sample AI teaching resources provided by the Teaching, Learning, and Professional Development Center at Texas Tech University (https://www.depts.ttu.edu/tlpdc/AI_Resources/AIResources.php).
Institutions must also provide resources to support different constituencies learning about and using AI (Figure 3). Resources may include faculty development programs that equip instructors with the knowledge and skills to effectively teach AI-related content, as well as student resources that promote digital literacy and ethical AI use. The availability of these resources will determine the extent to which students and faculty can engage meaningfully with AI in education, including UX pedagogy. College and department-level administrations need to promote transparency and accountability with AI applications, ensuring that AI tools are used in ways that align with UX professional values.
More on Ethics
Ethics is a core component of UX education. UX educators are responsible for preparing students for the ethical challenges they will face in their professional lives. Educators must encourage students to consider the broader societal implications of their work. One major ethical concern is the potential for AI to exacerbate existing inequalities and biases. AI systems are often trained on biased data, which can result in discriminatory outcomes. UX design students must be aware of these risks and learn to take steps to mitigate the risks in their work. They need to practice advocating for diverse and representative data sets, conducting thorough testing for bias, and promoting transparency in AI-driven systems, as Carol Smith (2019) noted in a JUX issue.
Another ethical consideration is the impact of AI on privacy and security. As AI systems become more integrated into everyday life, they collect and process vast amounts of personal data. UX designers must consider the implications of this data collection and ensure that users’ privacy is protected. UX requires a commitment to data ethics to design secure and user-friendly systems (Obrenovic et al., 2024). UX educators must prepare students to understand the ethical standards of their industry, advocate for responsible AI usage, and make informed decisions about the integration of AI into their work. By instilling a solid ethical foundation, we can trust that students will navigate the challenges of AI in alignment with their values and promote positive outcomes for users and society.
Four Scenarios
Recently, I participated in a collaborative autoethnographic study in which a group of communication instructors discussed about their approach toward AI in their teaching. As mentioned, we consulted Vinchon et al. (2023, pp. 476–477) for four scenarios that became the basis of our reflection (truncated here):
Case 1. Co-cre-AI-tion: A real collaborative effort involving more or less equally the human and the generative AI, with recognition of the contributions of each party. This can be called augmented creativity because the output is the result of a hybridization that would not be possible by humans or AI alone. This collaboration is considered the optimal future, …and it is starting to be a common position among researchers studying the possibilities offered by AI.
Case 2. Organic: This is creation by a human for humans, old-fashioned creativity. This pure human creativity will become a mark of value attributed to the works. …Similarly to how automation has resulted in mass production of goods and a reduction in artisan work, AI will replace a large portion of the jobs that can be automated. However, as with traditional craftsmanship, this might increase the perceived product quality, uniqueness, and authenticity of non-AI-supported creative output, which might become more attractive, called the handmade effect.
Case 3. Plagiarism 3.0: People desiring to appear productive and creative will draw heavily on AI productions without citing the source. …it emphasizes the legal debate on the content created by [AI], requiring trial and error to properly represent what the author has in mind.
Case 4. Shut down: This scenario posits that some people will become less motivated to conduct creative action at all. AI generates content based on existing sources, and these sources are a mash-up, a mixture of existing content previously generated by humans and then fed to the AI system in a training phase. In this scenario, some people will feel they are not able to create at the same level as AI and, thus, outsource the creation of content to generative AI.
I found this reflective exercise, in particular, motivated me to think about my own guiding principles in UX pedagogy.
For the first scenario, co-creation (Co-cre-AI-tion), I consider my own attitude toward generative AI’s place in our work and pedagogy to align with the premise of augmented creativity. In my own experimentation and use of AI for writing and research, I aim to achieve “a real collaborative effort” (Vinchon et al., 2023) so there is no exploitation of AI’s function in my work. I am also reminded of my university and department’s narratives about AI adoption in a way that represents an optimal future in which students, staff, and faculty members benefit optimally from the availability of AI—as we do with computers—rather than seeing it as a threat. The kind of co-creation I observe in current efforts undertaken by institutional units like our teaching and learning center, student conduct center, humanities center, and university library strikes a positive tone.
Some faculty members I met through local workshops have elected to keep AI at bay for their courses (for instance, calculus and music composition). This approach seems to fall under the Organic scenario, in which pure human creativity is valued over AI-supported output. For them, AI hinders the student’s learning of certain methods or processes that necessitate cognitive and behavioral engagement at a level that is uninterrupted by machine. Unlike the case of the Organic scenario, however, in which priority is placed on quality, uniqueness, and authenticity, the main objective of restricting AI use is to practice the human’s capacity to think—to observe a situation, detect and define a potential issue, identify sources of influence, and calculate possible outcomes—before taking actions accordingly. The concern here is about the extent to which human intelligence is exercised before artificial or augmentative tools are employed to aid in the thinking process. The rhetoric of authenticity is not appraised as a virtue here.
Rather, the notion of virtue (integrity) is a concern for some faculty members who worry most about Plagiarism 3.0, the scenario in which the ethical character of a product is superior. At a recent professional development session where I was asked to discuss my attitude and approaches to using AI in teaching, some faculty members in the audience outright spoke against AI as a writing and learning tool. For these faculty members, trust cannot be given to students without any consequence for violation of that trust. If given the opportunity, students will “draw heavily on AI productions without citing the source” (Vinchon et al., 2023), these faculty members contended. Thus, they think a clear policy must be installed to penalize students who will likely cheat using AI. This scenario upsets me as an instructor because it resides on the premise of policing students and instilling fear. If universities and instructors resort to this approach, students will never be able to use existing AI resources meaningfully or to innovate.
The last scenario, Shut down (or rather, move on), is what I observe to be the most likely scenario in the near future. Since last year, I have spoken to a few non-academics about professional use of AI in different workplace contexts, and to no surprise, they are less concerned about student academic integrity and more about job readiness. Based on previous experience with the hype of social media, big data, MOOCs (massive open online courses), wearable technology, and the Internet of Things, I am convinced that generative AI is the next disruptive technology to enter the “trough of disillusionment” (as in Gartner’s hype cycle) (Perri, 2023). This might look like accepting generative AI as an everyday technology that requires additional cognitive and physical labor to engage, which may result in a lack of interest or investment over time. Universities will likely retire their AI-specific policies or fold them into general academic integrity statements. Instructors will avoid AI unless the course specifically requires it (like in computer science). A few scholars in our field and adjacent will continue to investigate AI as posthuman agents in design, writing, and communication, but they will likely evolve to include the next disruptive technology appearing on the horizon.
So, Moving On
The final scenario could also be one in which AI becomes a normalized part of UX education and practice, in which it is possible that:
- Students are encouraged to experiment with AI tools, using them to generate ideas, explore design possibilities, and enhance their creative process. They use AI to support, rather than replace, human creativity, pushing the boundaries of what is possible in UX design.
- Educators incorporate real-world examples that highlight the ethical challenges of AI in UX design. Students are encouraged to critically evaluate AI-driven systems, considering the potential impact on marginalized communities and advocating for ethical practices in their work.
- Educators balance the integration of AI with the core principles of UX design, ensuring that students are equipped to navigate the complexities of AI while maintaining a human-centered perspective.
I close with this sentiment: UX and its training have always been dynamic, responding to the rapid advancements in technology. As we navigate the current and forthcoming landscape involving AI, it is essential to maintain a focus on the values that underpin UX education—empathy, ethics, and user-centered design—while embracing the possibilities that AI offers for innovation and creativity. Looking forward, I plan to incorporate more AI case studies and practical user scenarios into my UX courses. These case studies will provide students with opportunities to explore the use of AI in real-world contexts, allowing them to develop the language and skills needed to navigate AI-driven tools in the workplace.
References
Doukopoulos, L. (2024, July 2). Now more than ever, job of a faculty member is to design experiences that lead to learning. Chronicle of Higher Education. https://www.chronicle.com/blogs/letters/now-more-than-ever-job-of-a-faculty-member-is-to-design-experiences-that-lead-to-learning
Hocutt, D. (2024). Composing with generative AI on digital advertising platforms. Computers and Composition, 71, 102829. https://doi.org/10.1016/j.compcom.2024.102829
Kalpokienè, J. (2024). Law, human creativity, and generative artificial intelligence. Routledge.
Noy, S., & Zhang, W. (2023). Experimental evidence on the productivity effects of generative artificial intelligence. Science, 381(6654), 187–192. https://www.science.org/doi/full/10.1126/science.adh2586
Obrenovic, B., Gu, X., Wang, G., Godinic, D., & Jakhongirov, I. (2024). Generative AI and human–robot interaction: Implications and future agenda for business, society and ethics. AI & Society, 1–14. https://doi.org/10.1007/s00146-024-01889-0
Ooi, K. B., Tan, G. W. H., Al-Emran, M., Al-Sharafi, M. A., Capatina, A., Chakraborty, A., Dwivedi, Y. K., Huang, T.-L., Kar, A. K., Lee, V.-H., Loh, X.-M., Micu, A., Mikalef, P., Mogaji, E., Pandey, N., Raman, R., Rana, N. P., Sarker, P., Sharma, A., Teng, C.-I., Wamba, S. F., & Wong, L. W. (2023). The potential of generative artificial intelligence across disciplines: Perspectives and future directions. Journal of Computer Information Systems, 1–32. https://doi.org/10.1080/08874417.2023.2261010
Perri, L. (2023). What’s new in artificial intelligence from the 2023 Gartner hype cycle. Gartner. https://www.gartner.com/en/articles/what-s-new-in-artificial-intelligence-from-the-2023-gartner-hype-cycle
Schriver, K. (2023). Is artificial intelligence coming for information designers’ jobs? Information Design Journal, 28(1), 1–6. https://doi.org/10.1075/idj.00017.sch
Smith, C. (2019). Intentionally ethical AI experiences. Journal of User Experience, 14(4), 181–186. https://uxpajournal.org/ethical-ai-experiences/
Tham, J. (2017). Wearable writing: Enriching student peer reviews with point-of-view video feedback using Google Glass. Journal of Technical Writing and Communication, 47(1), 22–55. https://doi.org/10.1177/0047281616641923
Tham, J. (2018). Interactivity in an age of immersive media: Seven dimensions for wearable technology, internet of things, and technical communication. Technical Communication, 65(1), 46–65. https://www.ingentaconnect.com/contentone/stc/tc/2018/00000065/00000001/art00006
Tham, J., Howard, T., & Verhulsdonck, G. (2024). UX writing: Designing user-centered content. Routledge.
Verhulsdonck, G., Weible, J., Stambler, D. M., Howard, T., & Tham, J. (2024). Incorporating human judgment in AI-assisted content development: The HEAT heuristic. Technical Communication, 71(3). https://doi.org/10.55177/tc286621 Vinchon, F., Lubart, T., Bartolotta, S., Gironnay, V., Botella, M., Bourgeois-Bougrine, S., Burkhardt, J.-M., Bonnardel, N., Corazza, G.E., Glăveanu, V., Hanchett Hanson, M., Ivcevic, Z., Karwowski, M., Kaufman, J.C., Okada, T., Reiter-Palmon, R., & Gaggioli, A. (2023). Artificial intelligence & creativity: A manifesto for collaboration. Journal of Creative Behavior, 57(4), 472–484. https://doi.org/10.1002/jocb.597